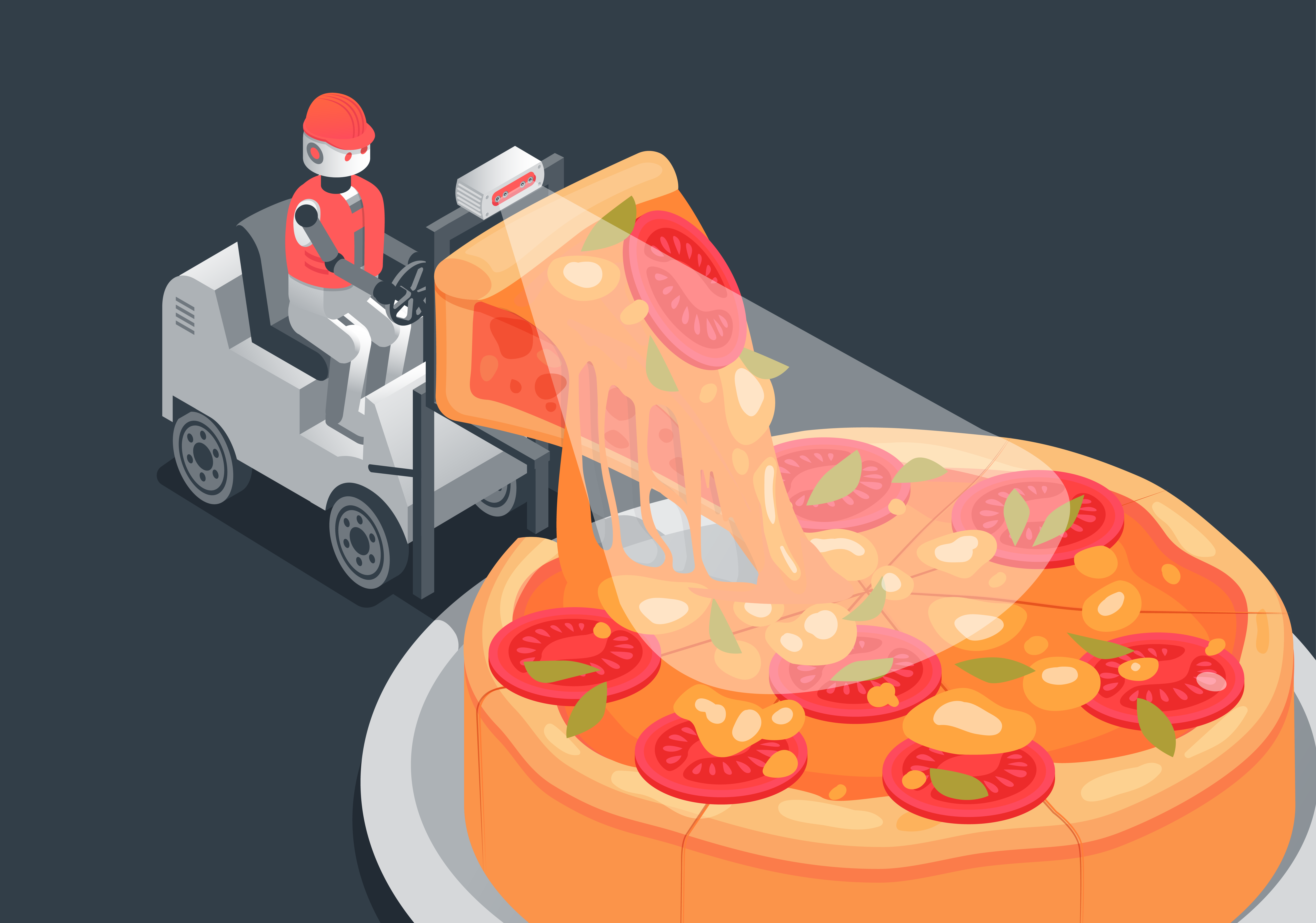
It was great to see ProMat back to full strength this year after the hiatus caused by COVID. In fact, it was a record-breaking event as over 1,050 exhibitors and nearly 51,000 visitors traveled to Chicago to see the latest in manufacturing and supply chain innovations. Of course, we were there to see, meet and discuss the latest trends in spatial intelligence in this critical sector. We came away convinced that there are immediate and significant opportunities in this vibrant sector and that our technology and approach can integrate simply and effectively with existing solutions to deliver real value, fast.
LIDAR + Vision
Robots, AMRs, AGVs, and increasingly ‘smart’ manually piloted intralogistics vehicles were everywhere at the conference. Manufacturing and logistics have been pioneers of these systems for years, and the COVID experience has only increased interest in and deployment of autonomous solutions. From purpose-built, completely automated pick-and-place warehouses to retro-fitting sensors to existing vehicle fleets for real-time logistics systems (RTLS), spatial intelligence was demonstrated in many forms and functions. What struck us was the opportunity to add vision to existing capabilities. For many, the localization challenge has been met, but our visual spatial intelligence can add another layer of situational awareness to improve operations in complex and fast-moving environments.
Efficiency and cost reductions were common goals for everyone at ProMat – whether owners or operators of large warehouses, major manufacturers, systems integrators, or robotics developers. Today’s manufacturing and logistics facilities are mixed environments where automated and manual machines work alongside people and various vehicles. Navigating extensive facilities with restricted and crowded spaces requires spatial awareness in three dimensions to stack and access goods in very narrow aisles, often three or more stories high. Orchestrating all these elements to work seamlessly and safely at speed is a challenge that goes beyond ensuring that each autonomous unit can locate itself in that space. RTLS systems help to choreograph the constant interaction of these elements to keep everything moving smoothly.
Maintaining this smooth dance of constant motion is where Slamcore’s algorithms can add value. LIDAR already does an excellent job of delivering cm-level accurate location and obstacle detection. Many devices also already include depth cameras for additional obstacle avoidance with a broader field of view than LIDAR. What we can do with our algorithms is take more information from those cameras to add new levels of intelligence that can improve the efficiency of individual machines and the system as a whole.
Knowing ‘What’ as well as ‘Where’ obstacles are
Our ‘Perceive’ level of spatial intelligence gives autonomous machines an added layer of situational awareness. Rather than just sensing an obstacle and stopping or slowing to avoid or navigate around it, we can identify what the obstacle is. AMRs can be programmed to react differently to different objects. Humans can be given a wider berth at a slower speed than other vehicles or pallets, for example. You can see our demo of this here. The overall average speed of an AMR is critical; avoiding lots of stop-start motion as robots encounter obstacles is a route toward far higher utilization and efficiency in carrying out tasks. One thing that vision can do to assist this is to confirm when obstructions are not there. For example, knowing that there are no humans in the immediate field of view can help increase average operational speeds. The more information on ‘what’ things are and ‘where’ they are, the fewer unnecessary stops or slowdowns.
Through our algorithms, vision can also help build a shared, real-time, dynamic map of an entire facility. Capturing and sharing detailed information about the ever-changing layout of a facility as goods, vehicles, and even shelf units move can improve the ability of RTLS to constantly optimize routes, speeds, and utilization of all elements of that system. Vision represents a rich language of situational awareness that can be easily shared between humans and machines. As every vehicle captures its view of the world around it, identifies what objects it sees, and shares these, better, more accurate, detailed, and useful maps are created. RTLS can use these to constantly optimize routes, deploying the right asset in the timeliest fashion.
Faster ROI
Best of all, working closely with existing LIDAR and onboard cameras, this new layer of situational awareness can be added quickly and cost-effectively. Slamcore algorithms are optimized to be memory and processor efficient and can run on existing hardware in many cases. Agreements with the leading silicon providers in the sector, including Nvidia, Texas Instruments, and Qualcomm, mean that our solutions are optimized, tried, and tested on the most common chips used by AMR and robot developers.
Return on Investment remains one of the biggest barriers to the wider deployment of autonomous solutions in manufacturing and logistics. Customers, although faced with increasingly pressing labour shortages, still need to see payback from investments in robots in around 18 months on average. We believe that adding Slamcore to these setups will have marginal impact on cost, but could accelerate that time to ROI significantly. The better the average speed, and thus the amount of work an AMR can accomplish, the more useful it is and the faster the ROI. Visual spatial intelligence improves the performance of every unit and creates system-wide situational awareness that supports further optimization.
We had many detailed and productive conversations at ProMat, and are excited to see our algorithms working alongside LIDAR SLAM to bring new capabilities and new value to this rapidly expanding market. If you want to hear more about how Slamcore can add perception and increase ROI, please get in touch.
Ends
🤖